Unlocking Efficiency: The Power of Auto Data Mapping Tools for a Data-Driven Enterprise
December 6th, 2023 WRITTEN BY Soumen Chakraborty, Director - Data Management Tags: AI, artificial intelligence, auto data mapping tools, data driven, data governance, data management, data modeling, Industry-agnostic, mapping
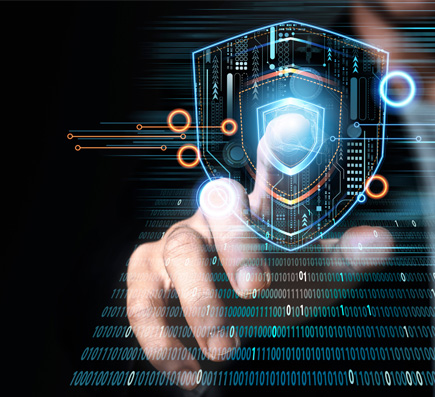
Written By Soumen Chakraborty and Vaibhav Sathe
In the fast-paced world of data-driven decision making, enterprises are constantly grappling with vast amounts of data scattered across diverse sources. Making sense of this data and ensuring its seamless integration is a challenge that many data teams face. Enter the hero of the hour: AI-Driven Auto Data Mapping Tools.
Understanding the Need:
Consider this scenario: Your enterprise relies on data from various departments – sales, marketing, finance, and more. Each department might use different terms, structures, and formats to store their data. Moreover, each company depends on a multitude of third-party data sources, over which they often have minimal to no control. Manual mapping of these diverse datasets is not only time-consuming but also resource intensive, costly, and prone to errors.
Traditional data mapping tools offer some automation, but they highly depend on the tool user’s skill set. However, the modern auto data mapping tools take it a step further. They leverage advanced algorithms to analyze not just data fields but also data, metadata, context, and semantics. This comprehensive approach ensures a deeper understanding of the data, resulting in more accurate and contextually relevant mappings.
How it helps?
- Precise Mapping:
There is a high chance of human error, especially when dealing with large datasets. Auto data mapping tools excel at recognizing intricate patterns within datasets. Whether it is identifying synonyms, acronyms, or variations in data representations, these tools analyze the nuances to provide precise mappings. Thus, auto data mapping tools significantly reduce the risk of mistakes in data mapping, ensuring that your reports and analytics are based on accurate information.
Practical Example: In a healthcare dataset, where “DOB” may represent both “Date of Birth” and “Date of Admission,” an auto data mapping tool can discern the semantics and map each instance accurately.
It can also automate the process of linking data fields and relationships. For instance, your marketing team uses “CustomerID,” while the finance team refers to it as “ClientID” and some other team identifies it as “Account Number.” An auto data mapping tool can recognize these connections, eliminating the need for tedious manual matching.
- Accelerated Data Modeling:
In a traditional data modeling approach, data analysts manually analyze each dataset, identify relevant fields, and establish relationships. This process is time-consuming and prone to errors, especially as datasets grow in complexity.
With auto data mapping, advanced algorithms can analyze datasets swiftly, recognizing patterns and relationships automatically. it can have the capability to potentially anticipate the relationships and logical modeling required for integrating a new data source with the existing dataset.
Practical Example:
Consider a scenario where the retail company introduces a new dataset related to online customer reviews. Without auto data mapping, analysts would need to manually identify how this new dataset connects with existing datasets. However, with auto data mapping, the tool can predict relationships by recognizing common attributes such as customer IDs or product codes. This accelerates the data modeling process, allowing analysts to quickly integrate the new dataset into the existing data model without extensive manual intervention.
- Adapting to Change:
In the dynamic business landscape, changes in data structures are inevitable. When a new department comes on board or an existing one modifies its data format, auto data mapping tools automatically adjust to these changes. It’s like having a flexible assistant that effortlessly keeps up with your evolving data needs.
Practical Example: Imagine your company acquires a new software system with a different data format. A reliable auto data mapping tool can seamlessly integrate this new data source without requiring a complete overhaul of your existing mapping by predicting the new mapping dynamically.
- Collaboration Made Easy:
Data teams often work in silos, each with its own set of terminology and structures. Auto data mapping tools create a common ground by providing a standardized approach to data mapping. This not only fosters collaboration but also ensures that everyone is on the same page, speaking the same data language.
Practical Example: In a collaborative environment, such tool can enable data SMEs from different departments to share insights and collectively refine semantic mappings, debate/define standards, promoting a shared understanding of data across the organization.
- Mapping Version Control:
Auto data mapping tools introduce mapping version control features, allowing data teams to track changes, revert to previous versions, and maintain a clear history of mapping modifications. This is invaluable to collaborative environments where multiple stakeholders contribute to data mapping.
In a dynamic data environment, where frequent updates and changes occur, mapping version control becomes crucial. Auto data mapping tools can provide the necessary systematic approach to Source-To-Target mapping versioning, ensuring transparency and collaboration among data teams.
Practical Example:
Such a tool can do precise tracking of mapping changes over time, offering a clear history of modifications with details about the user responsible and the purpose behind each mapping. In scenarios where unintended changes occur, the ability to easily revert to previous versions can ensure swift restoration of accurate data mappings, minimizing disruptions. Collaborative workflows are significantly enhanced, as multiple team members can concurrently work on different aspects of the mapping, with the tool seamlessly managing the merging of changes. Moreover, the audit trail provided by the version control tool can contribute to efficient compliance management, offering transparency and demonstrating adherence to data governance standards.
- Compliance and Governance:
In an era of data regulations, ensuring compliance is non-negotiable. Auto data mapping tools contribute to data governance efforts by providing transparency into how data is mapped and transformed. This transparency is crucial for audits and compliance checks.
Practical Example: Consider a scenario where your industry faces new data privacy regulations. An auto data mapping tool can help you quickly identify and update mappings that are needed to comply with the new rules, ensuring your organization stays within legal boundaries.
- Cost Reduction:
Manual data mapping is resource intensive. Auto data mapping tool can streamline the integration process, saving time and resources. This efficiency translates to cost savings for your enterprise.
Practical Example: Imagine the person-hours saved when your data team does not have to manually reconfigure mappings every time a new dataset is added.
- Improved Decision Making:
A clear understanding of data relationships is crucial for effective decision making. Understanding the context in which data is used is crucial for effective integration. Auto-Data Mapping tools take into account the broader context of data fields, ensuring that mappings align with the intended use and purpose. Auto data mapping tools provide this clarity, empowering data analysts and scientists to work with well-organized and accurately mapped data.
Practical Example: Consider a sales dataset where “Revenue” may be reported at both the product and regional levels. An auto data mapping tool can discern the context, mapping the data based on its relevance to specific reporting requirements.
With accurate data mappings, your business intelligence team can confidently create reports and analysis that the leadership can trust, leading to more informed decisions.
What tools to use?
Despite the numerous benefits of auto data mapping, there is a notable shortage of effective tools in the industry. This is primarily due to a lack of awareness regarding the needs and implications of having or not having such a tool. Additionally, there is a prevailing notion that ETL tools/developers can adequately address these requirements, leading to a lack of interest in dedicated data mapping tools. However, this should not be the optimal solution for today’s data-driven organizations.
Building data plumbing without proper data mapping is like constructing a house without a blueprint—it just doesn’t work! Data Mapping, being both functional metadata and a prerequisite for creating accurate data integration pipelines, should be crafted, and handled independently. Otherwise, there is a potential risk of losing vital information concealed within diverse standalone data integration pipelines. Organizations often pay a hefty price by not maintaining separate mapping of source to target outside the code. It causes a lack of awareness of lineage and makes real-time monitoring or modern needs like data observability almost impossible, because nobody knows what is happening in those pipelines without decoding the entire pipeline.
With this consideration in mind, Fresh Gravity has crafted a tool named Penguin, a comprehensive AI-driven data matcher and mapper tool that helps enterprises define and create a uniform and consistent global schema from heterogeneous data sources. A clever data mapping tool that not only matches the abilities of auto data mapping tools but also brings in a sharp industry focus, adaptive learning with industry smarts, and collaborative intelligence to supercharge data integration efforts. For companies handling intricate data and numerous data integration pipelines, leveraging a tool like Penguin alongside a metadata-driven data integration framework is crucial for maximizing the benefits of automated data integration. It makes creating maps easy, helps teams work together smoothly, and keeps track of changes.
In conclusion, auto data mapping tools are indispensable for modern enterprises seeking to navigate the complex landscape of data integration. By enhancing efficiency, accelerating data modeling, ensuring accuracy, fostering collaboration, and facilitating compliance, these tools pave the way for organizations to derive maximum value from their data. Fresh Gravity’s dedication to excellence in these areas makes our tool valuable for succeeding with data. So, embrace the power of automation, and watch your enterprise thrive in the era of data excellence.
If you would like to know more about our auto data mapping tool, Penguin, please feel free to write to us @ info@freshgravity.com.