Data Observability is the new Data Quality – What, Why and How ?
June 6th, 2023 WRITTEN BY Soumen Chakraborty, Director - Data Management Tags: analyse data, Data, data management, data monitoring, decisions, Industry-agnostic, observability
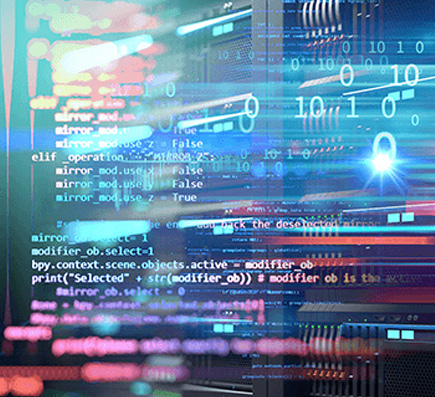
Written By : Soumen Chakraborty and Vaibhav Sathe
In today’s data-driven world, organizations are relying more and more on data to make informed decisions. With the increasing volume, velocity, and variety of data, ensuring data quality has become a critical aspect of data management. However, as data pipelines become more complex and dynamic, traditional data quality practices are no longer enough. This is where data observability comes into play. In this blog post, we will explore what data observability is, why it is important, and how to implement it.
What is Data Observability?
Data observability is a set of practices that enable data teams to monitor and track the health and performance of their data pipelines in real time. This includes tracking metrics such as data completeness, accuracy, consistency, latency, throughput, and error rates. Data observability tools and platforms allow organizations to monitor and analyze data pipeline performance, identify, and resolve issues quickly, and improve the reliability and usefulness of their data.
The concept of data observability comes from the field of software engineering, where it is used to monitor and debug complex software systems. In data management, data observability is an extension of traditional data quality practices, with a greater emphasis on real-time monitoring and alerting. It is a proactive approach to data quality that focuses on identifying and addressing issues as they occur, rather than waiting until data quality problems are discovered downstream.
Why is Data Observability important?
Data observability is becoming increasingly important as organizations rely more on data to make critical decisions. With data pipelines becoming more complex and dynamic, ensuring data quality can be a challenging task. Traditional data quality practices, such as data profiling and data cleansing, are still important, but they are no longer sufficient.
Let’s consider an example to understand why data observability is needed over traditional data quality practices. Imagine a company that relies on a data pipeline to process and analyze customer data. The data pipeline consists of multiple stages: extraction, transformation, and loading into a data warehouse. The company has implemented traditional data quality practices, such as data profiling and data cleansing, to ensure data quality.
However, one day the company’s marketing team notices that some of the customer data is missing in their analysis. The team investigates and discovers that the data pipeline had a connectivity issue, which caused some data to be dropped during the transformation stage. The traditional data quality practices did not catch this issue, as they only checked the data after it was loaded into the data warehouse.
With data observability, the company could have detected the connectivity issue in real time and fixed it before any data was lost. By monitoring data pipeline performance in real-time, data observability can help organizations identify and resolve issues quickly, reducing the risk of data-related errors and improving overall data pipeline performance.
In this example, traditional data quality practices were not sufficient to detect the connectivity issue, highlighting the importance of implementing data observability to ensure the health and performance of data pipelines.
Data observability provides organizations with real-time insights into the health and performance of their data pipelines. This allows organizations to identify and resolve issues quickly, reducing the risk of data-related errors and improving the reliability and usefulness of their data. With data observability, organizations can make more informed decisions based on high-quality data.
How to Implement Data Observability ?
Implementing data observability requires a combination of technology and process changes. Here are some key steps to follow:
Define Metrics: Start by defining the metrics that you want to track. This could include metrics related to data quality, such as completeness, accuracy, and consistency, as well as metrics related to data pipeline performance, such as throughput, latency, and error rates.
Choose Tools: Choose the right tools to help you monitor and track these metrics. This could include data quality tools, monitoring tools, or observability platforms.
Monitor Data: Use these tools to monitor the behavior and performance of data pipelines in real time. This will help you to identify and resolve issues quickly.
Analyze Data: Analyze the data that you are collecting to identify trends and patterns. This can help you to identify potential issues before they become problems.
Act: Finally, take action based on the insights that you have gained from your monitoring and analysis. This could include making changes to your data pipeline or addressing issues with specific data sources.
Benefits of Data Observability
Implementing data observability provides numerous benefits, including:
Improved Data Quality: By monitoring data pipeline performance in real time, organizations can quickly identify and address data quality issues, improving the reliability and usefulness of their data.
Faster Issue Resolution: With real-time monitoring and alerting, organizations can identify and resolve data pipeline issues quickly, reducing the risk of data-related errors and improving overall data pipeline performance.
Better Decision Making: With high-quality data, organizations can make more informed decisions, leading to improved business outcomes.
Increased Efficiency: By identifying and addressing data pipeline issues quickly, organizations can reduce the time and effort required to manage data pipelines, increasing overall efficiency.
Data observability is a new concept that is becoming increasingly important in the field of data management. By providing real-time monitoring and alerting of data pipelines, data observability can help to ensure the quality, reliability, and usefulness of data. Implementing data observability requires a combination of technology and process changes, but the benefits are significant and can help organizations to make better decisions based on high-quality data.