Leading Pharmaceutical Company
September 20th, 2024 WRITTEN BY Fresh Gravity Tags: Analytics & ML, Life Sciences
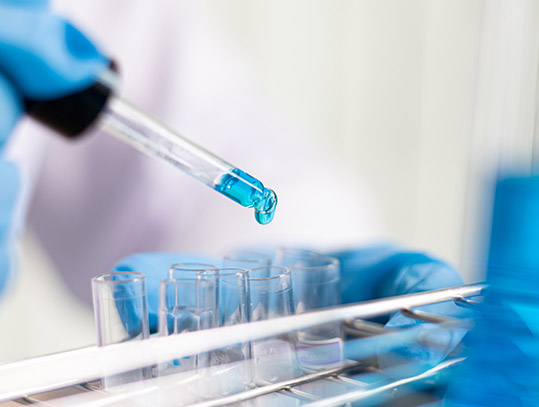
Fresh Gravity implemented a solution leveraging two NLP algorithms: TD-IDF and BERT. This helped in accurately identifying more than 95% of pregnancy cases in the production environment.
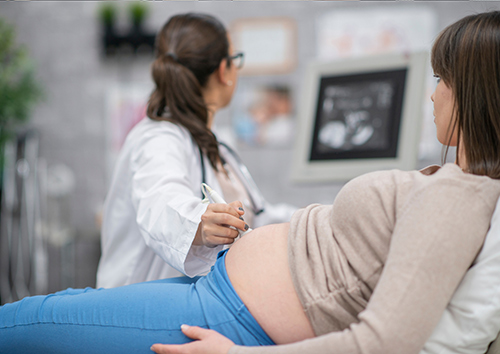
Problem
The client needed an automated approach to validate pregnancy cases to improve the accuracy of their reporting to regulatory authorities. They used three different fields to manually denote that a case is pregnancy related.
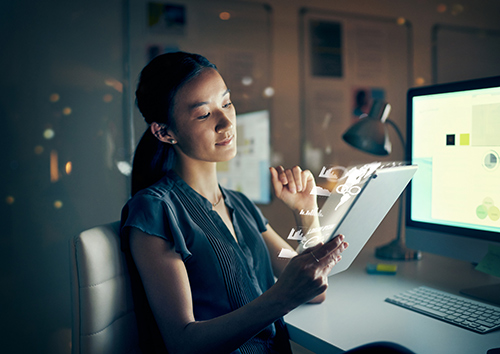
Solution
Fresh Gravity solved the problem by leveraging two Natural Language Processing (NLP) algorithms: TD-IDF and BERT. Our team applied TF-IDF to known pregnancy cases to develop a list of terms that are frequently used. Bidirectional Encoder Representations from Transformers is a technique for NLP that is pretrained by Google. This cutting-edge algorithm is trained to have a deeper sense of context while improving prior NLP models that read text in a single direction.
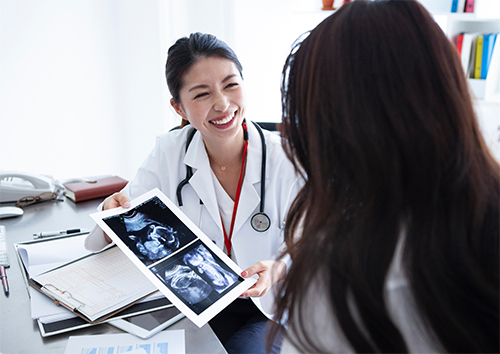
Impact
This model accurately identified more than 95% of pregnancy cases in production environment also improved the quality of client’s Pharmacovigilance process. The algorithm enabled the client to automatically detect and ensure all cases containing pregnancy-related terms can be marked appropriately.